LMQL
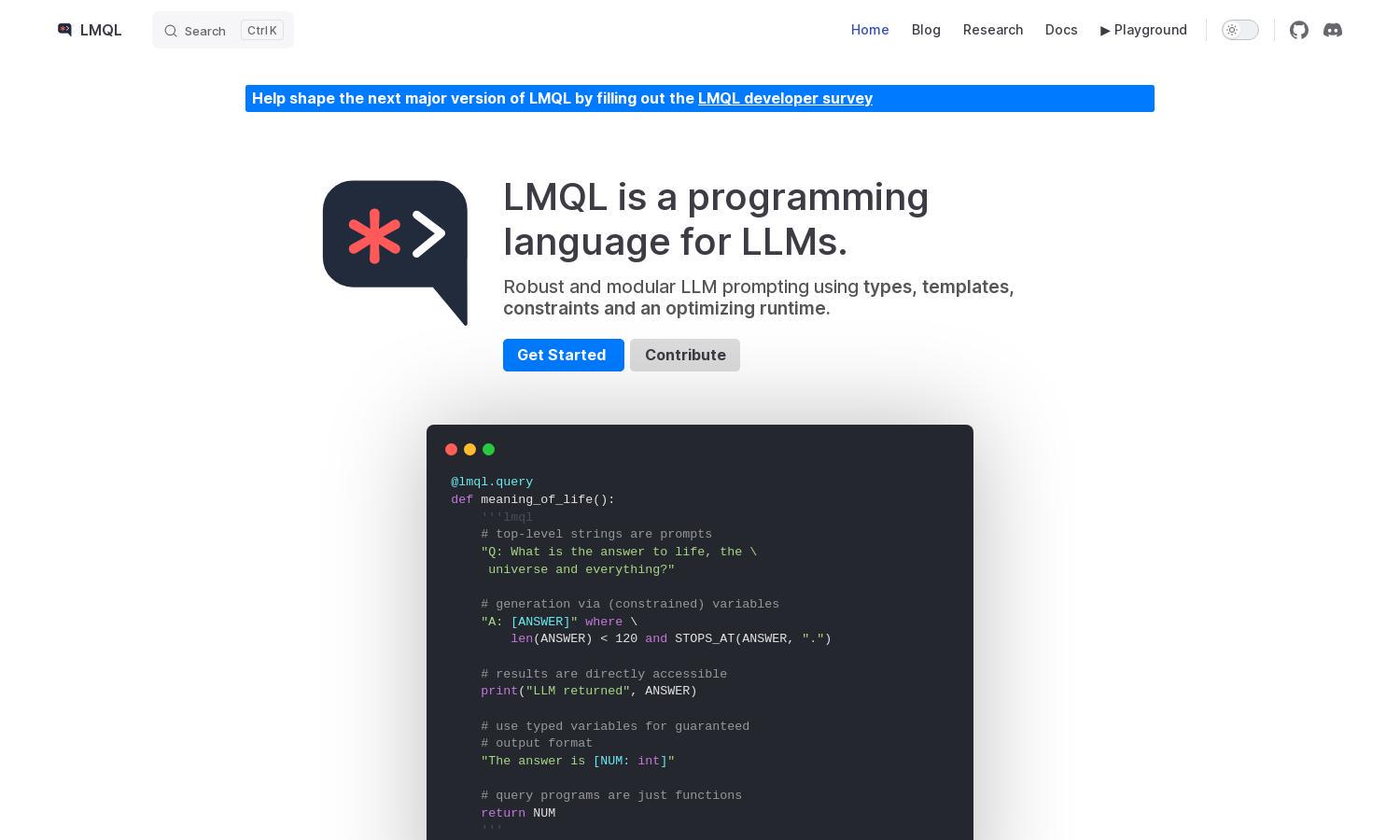
About LMQL
LMQL is an innovative programming language for Large Language Models (LLMs), aimed at developers wanting to streamline interactions with AI systems. Its unique modular query structure allows users to create reusable and efficient prompts, enhancing productivity while offering a robust framework for advanced AI applications.
LMQL offers a range of pricing plans tailored to developers, ensuring affordability. Basic access is free, while premium subscriptions include additional features like advanced templates and backend portability. Upgrading provides enhanced capabilities, making LMQL a smart choice for serious developers navigating LLM complexities.
The user interface of LMQL is designed for simplicity and efficiency, focusing on delivering a seamless interaction experience with LLMs. With intuitive layouts and easy navigation, users can quickly access features, making it a valuable tool for both novice programmers and experienced developers.
How LMQL works
Users start by onboarding through a straightforward setup on LMQL, creating modular queries for LLMs. The platform allows seamless integration of Python code, enabling personalized prompt generation and efficient management of responses. With its optimizing runtime, LMQL ensures that developers can effortlessly adjust their queries.
Key Features for LMQL
Modular Queries
LMQL’s modular query feature allows users to create reusable prompt components, enhancing prompt construction and response management. This unique capability benefits developers by streamlining the process of interacting with LLMs and improving the overall efficiency of programming tasks.
Portability Across Backends
LMQL supports easy switching between various LLM backends with just one line of code, significantly enhancing user flexibility. This portability feature allows developers to choose the best backend for their needs without extensive code modifications, making LMQL highly adaptable for diverse applications.
Typed Variables
LMQL introduces typed variables that guarantee output formats when interacting with LLMs. This feature enhances precision and predictability, allowing developers to structure their queries effectively and ensuring that the model’s responses adhere to predefined formats.